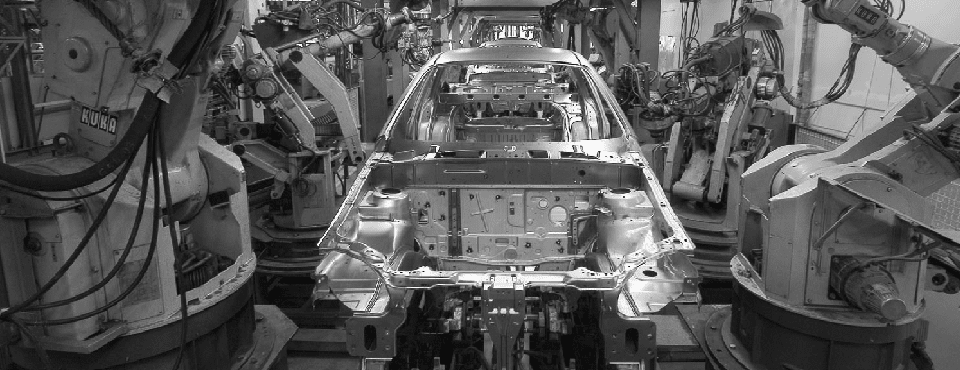
Industrial robots on workers: Winners and losers
Repost from VoxEU.org.
Theory suggests that ‘directly affected’ workers who perform substitutable tasks, such as those employed in blue-collar, routine occupations, are more likely to be losers from robot adoption, while the productivity gains these machines bring could benefit workers performing complementary tasks. But there is surprisingly little evidence on this first-order protection. This column uses data from the Netherlands to show that directly affected workers face lower earnings and employment rates, while other workers indirectly gain from robot adoption. This is true both for workers employed in robot-adopting firms and their competitors.
According to the International Standards Organization, an industrial robot is an actuated mechanism programmable in two or more axes, with a degree of autonomy, moving within its environment, to perform intended tasks. Industrial robots have already automated a variety of production tasks, from painting to welding, sorting and assembling, and they are on the rise. In the US, for example, the number of robots per 10,000 industrial workers increased from 35 in 1993 to 149 in 2014 and to 255 in 2020. The increase has been even starker in the Netherlands, where the number of robots per thousand industrial workers increased from 12 in 1993 to 209 in 2020.
Previous Vox columns and a burgeoning literature recognise that automation should have heterogeneous impacts on workers. For example, Golin and Rauh (2023) indicate that perceived automation risk strongly relates to workers’ preferences for redistribution and employment responses. Prettner and Bloom (2020) point out that robots mainly replace the routine tasks of low-skilled workers, while wealthier capital owners are likely the beneficiaries. The fact that industrial robots particularly affect routine and low-skilled workers is one of the reasons why Rebelo et al. (2020) advocate a robot tax, at least in the short run, as a way to protect workers who cannot acquire non-routine skills. These perspectives notwithstanding, there is little empirical evidence on whether industrial robots indeed negatively impact certain groups of workers – and whether some other types of workers simultaneously benefit.
In a recent paper, we study the effects of the adoption of industrial robots on workers in the Netherlands (Acemoglu et al. 2023). The Dutch economy provides an interesting context. In addition to being at the forefront of the spread of industrial robotics, as the numbers provided above suggest, the Dutch labour market has extensive labour market regulations that are meant to protect workers against disruptions and job loss. Lessons from the Dutch context can thus inform us both on the heterogeneous effects of robot adoption and on whether labour market regulations can successfully shield the most affected workers from negative consequences.
We use data from the trade register to obtain reliable information on robot adoption by tracking imports of manufacturing firms. We combine this with high-quality matched employer-employee data, covering about a million Dutch workers in manufacturing between 2009 and 2020.
Our results first confirm that the firm-level effects of robot adoption are similar in the Netherlands to those in other industrialised economies. Employment and value-added increase and labour share declines at robot-adopting firms. This overall pattern and the quantitative magnitudes of our estimates are very similar to those presented in Acemoglu et al. (2020) for France and Koch et al. (2021) for Spain. Moreover, as in French and Spanish manufacturing, these positive effects among adopters are only one side of the coin. The other side are significant negative effects on their competitors in the same industry, which experience lower value added and employment. This pattern also raises questions as to whether the effects on workers will be heterogeneous not just by skill but also by whether they are employed at robot-adopting or non-adopting firms.
To explore these questions, we distinguish directly affected workers from those that are indirectly affected. Directly affected workers are those employed in tasks that can be performed by robots and thus face a risk of displacement (Acemoglu and Restrepo, 2020). Labour market implications of robot adoption depend not just on displacement but also on the productivity gains that they bring. These productivity effects imply that indirectly affected workers (who should not be displaced from their tasks) should benefit from robot adoption.
We construct three complementary measures of directly affected workers. The first is blue-collar workers employed in routine tasks — the ones in which robots are now most commonly employed. The second measure is based on the replaceability index of Graetz and Michaels (2018), and, similarly, captures workers in occupations that involve tasks that robots can perform. The third measure simply focuses on the highest completed level of education by a worker, since lower-education workers are more likely to specialise in manual tasks substitutable with robots.
The results are very similar with all three measures and also when we include worker fixed effects so as to trace impacts on the same workers over time. As shown in Figure 1, directly affected workers experience lower hourly wages after robot adoption, while those who are not directly impacted enjoyed higher earnings.
For example, in Panel A, without worker fixed effects, the wages of directly affected workers, in this instance measured as those performing blue-collar routine tasks, fall by about 6% following robot adoption, while indirectly affected workers enjoy wage gains of about 3.5%. The quantitative implications are somewhat smaller when worker fixed effects are included, presumably because this controls for worker selection. The results are similar for other measures of directly affected workers.
The negative employment and value-added changes at competitors suggest that to obtain a holistic picture of the worker-level implications of industrial robots, we also need to consider the impact on workers in other firms. When we do this, the patterns are broadly similar. Indirectly affected workers employed at competitors gain, while directly affected workers do not. These results suggest that not just opportunities at robot-adopting firms, but, relative to other workers, overall market opportunities deteriorate for workers specialized in tasks substitutable to industrial robots.
There are also heterogeneous effects on employment, though these are somewhat less precisely estimated, perhaps partly because of the slow adjustment in the heavily regulated Dutch labour market.
In sum, robot adoption appears to have highly heterogeneous effects on workers, in line with theoretical expectations. Whether we use the measure of workers performing blue-collar routine tasks or replaceable tasks, or focus on differences by worker education, directly affected workers seem to lose from robot adoption, while indirectly affected workers gain. These patterns thus reiterate the major distributional consequences of automation.
References
Acemoglu, D, H R A Koster and C Ozgen (2023), “Robots and workers: Evidence from the Netherlands”, NBER Working Paper 31009.
Acemoglu, D and P Restrepo (2020), “Robots and Jobs: Evidence from US labor markets”, Journal of Political Economy 128(6): 2188-2244.
Acemoglu, D, C Lelarge and P Restrepo (2020), “Competing with robots: Firm-level evidence from France”, AEA Papers and Proceedings 110: 383-388.
Golin, M and C Rauh (2023), “Workers’ responses to the threat of automation”, VoxEU.org, 8 March 8.
Graetz, G and G Michaels (2018), “Robots at work”, Review of Economics and Statistics 100(5): 753-768.
Koch, M, I Manuylov and M Smolka (2021), “Robots and firms”, Economic Journal 131(638): 2553-2584.
Prettner, K and D Bloom (2020), “The macroeconomic effects of automation and the role of COVID-19 in reinforcing their dynamics”, VoxEU.org, 25 June.
Rebelo, S, P Teles and J Guerreiro (2020), “Robots should be taxed, for a while”, VoxEU.org, 20 August.